Prescriptive Analytics
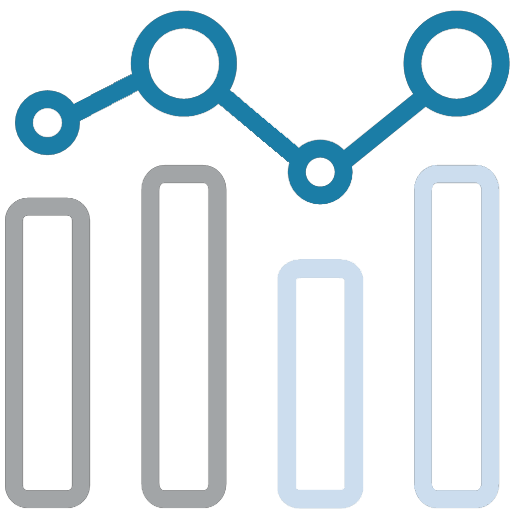
Prescriptive analytics goes beyond predicting future outcomes by recommending specific actions to achieve desired results. It combines data, algorithms, and business rules to provide actionable insights.
- Process:
- Data Preparation: Collect and clean data from relevant sources, including historical and real-time data.
- Model Development: Use advanced analytics techniques, such as optimization algorithms, simulation, and machine learning, to create models that recommend actions.
- Scenario Analysis: Test different scenarios to evaluate the potential outcomes of recommended actions.
- Actionable Recommendations: Provide clear and specific recommendations based on the analysis.
- Integration: Integrate prescriptive analytics into decision-making processes, such as supply chain management or marketing campaigns.
- Purpose:
The goal of prescriptive analytics is to enable data-driven decision-making by recommending the best course of action to achieve specific objectives. - Outcome:
Actionable recommendations that optimize outcomes, improve efficiency, and reduce risks. - Challenges:
Developing accurate and reliable prescriptive models requires expertise and high-quality data. Additionally, integrating recommendations into decision-making processes can be complex. - Best Practices:
- Use a combination of historical and real-time data to improve model accuracy.
- Test and validate models using real-world scenarios.
- Provide clear and actionable recommendations that align with business goals.
- Continuously update and refine models to reflect changes in the environment.